Despite significant advances in neuroscience over the recent years, the complexity of neural activity has defied efforts to formulate a comprehensive understanding of the human brain. Progress towards this goal has been hindered by technological and methodological constraints in accessing brain function, motivating recent efforts to develop and apply new technologies. A prominent barrier is the limitation of current non-invasive brain imaging technologies, which enable access to either high temporal information (MEG/EEG), high spatial information (fMRI), but not both simultaneously.
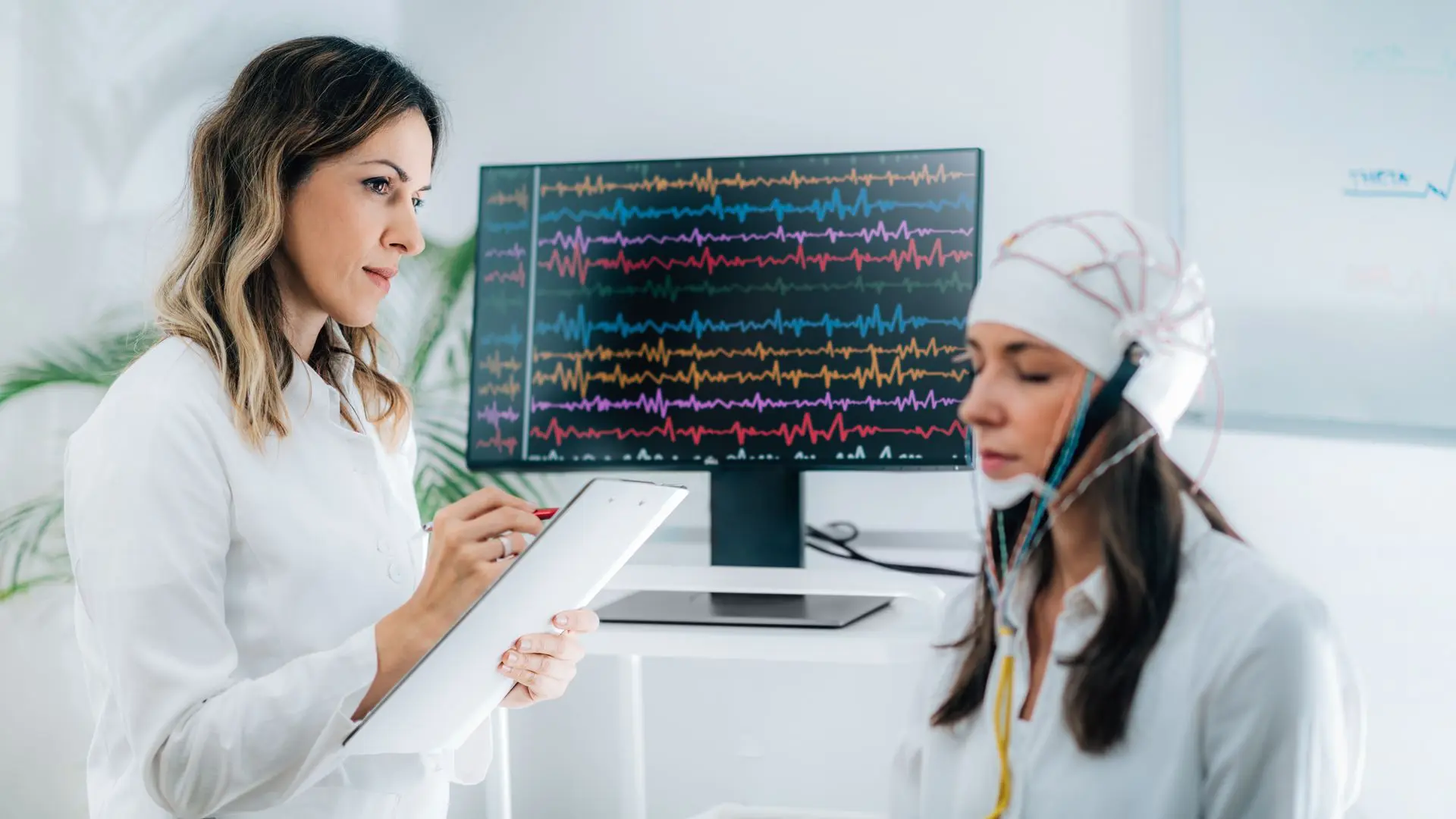
However, the field is now transforming: multivariate pattern analysis tools are ubiquitous in fMRI and are becoming increasingly popular in MEG/EEG. The introduction of modern machine learning algorithms to decipher information from ongoing neuronal processes has drastically improved the quality of extracted neural signals. This is the central theme of our research, which focuses on novel methodology for discerning neural representations from MEG data, and development of multimodal imaging techniques. The group follows the following main research lines:
Resolve the neural computations that transform low-level visual representations into semantic content
Bridge the gap between human and computer vision.
Develop novel neuroimaging methods to holistically capture the spatiotemporal and representational space of brain activation
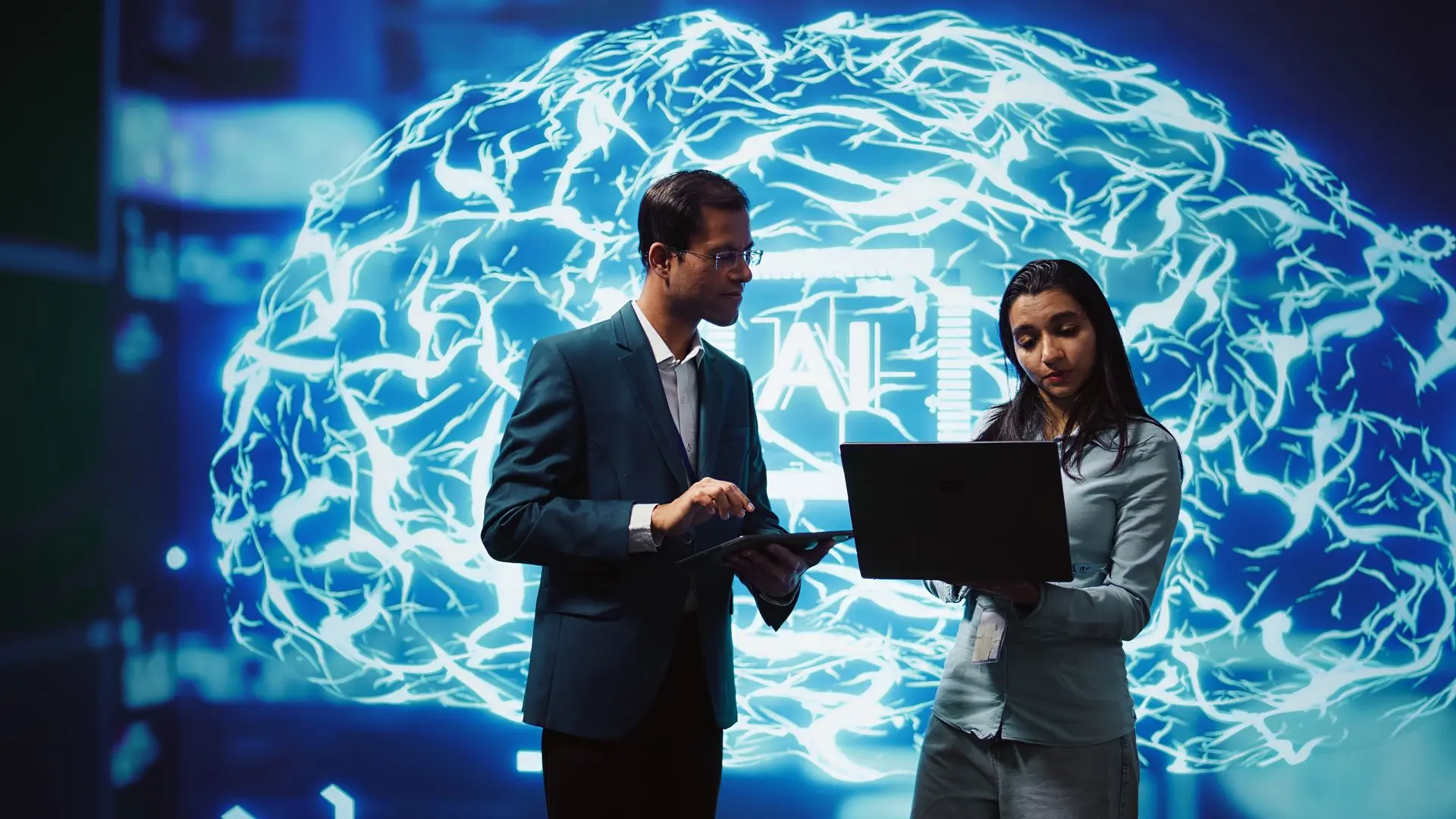
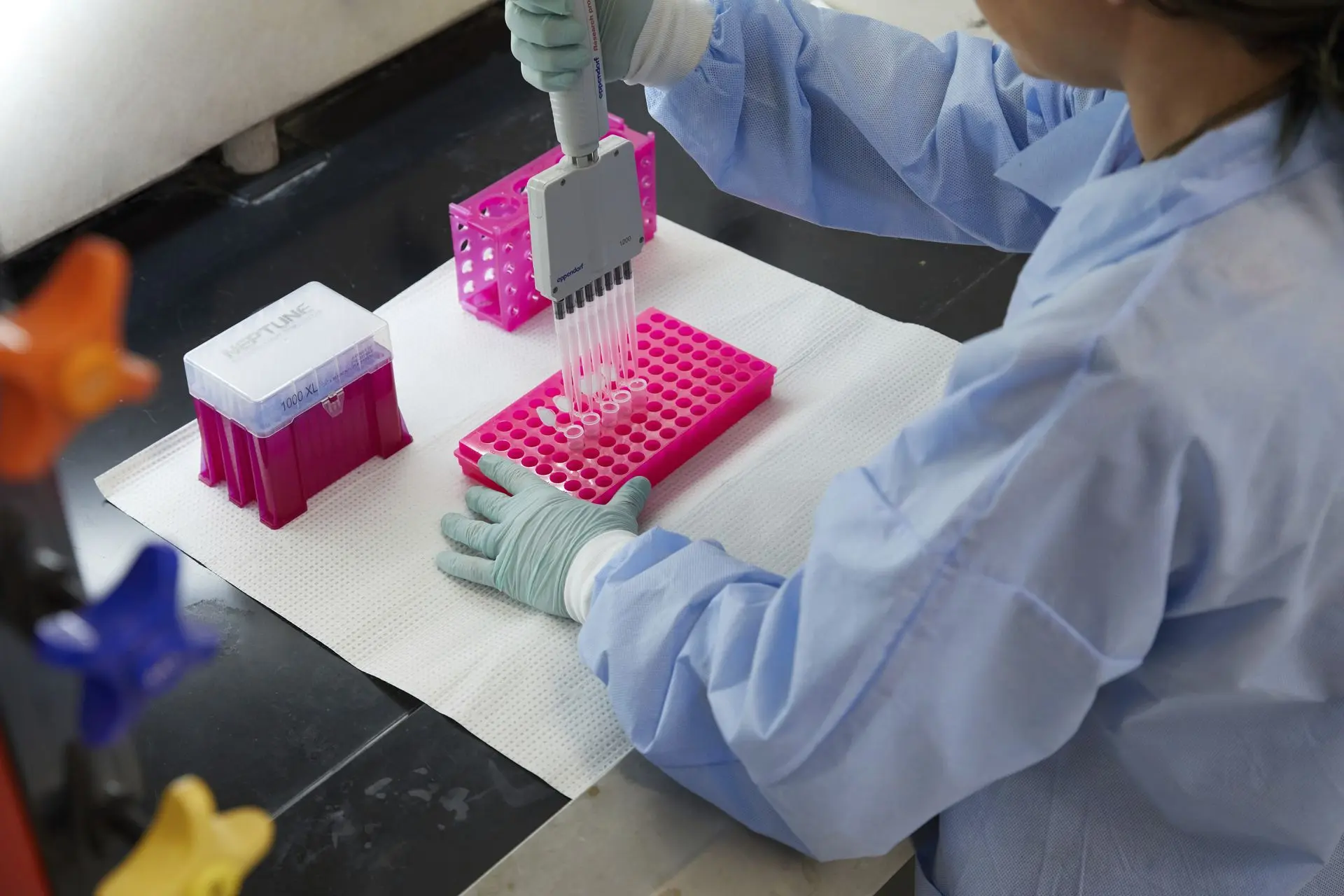
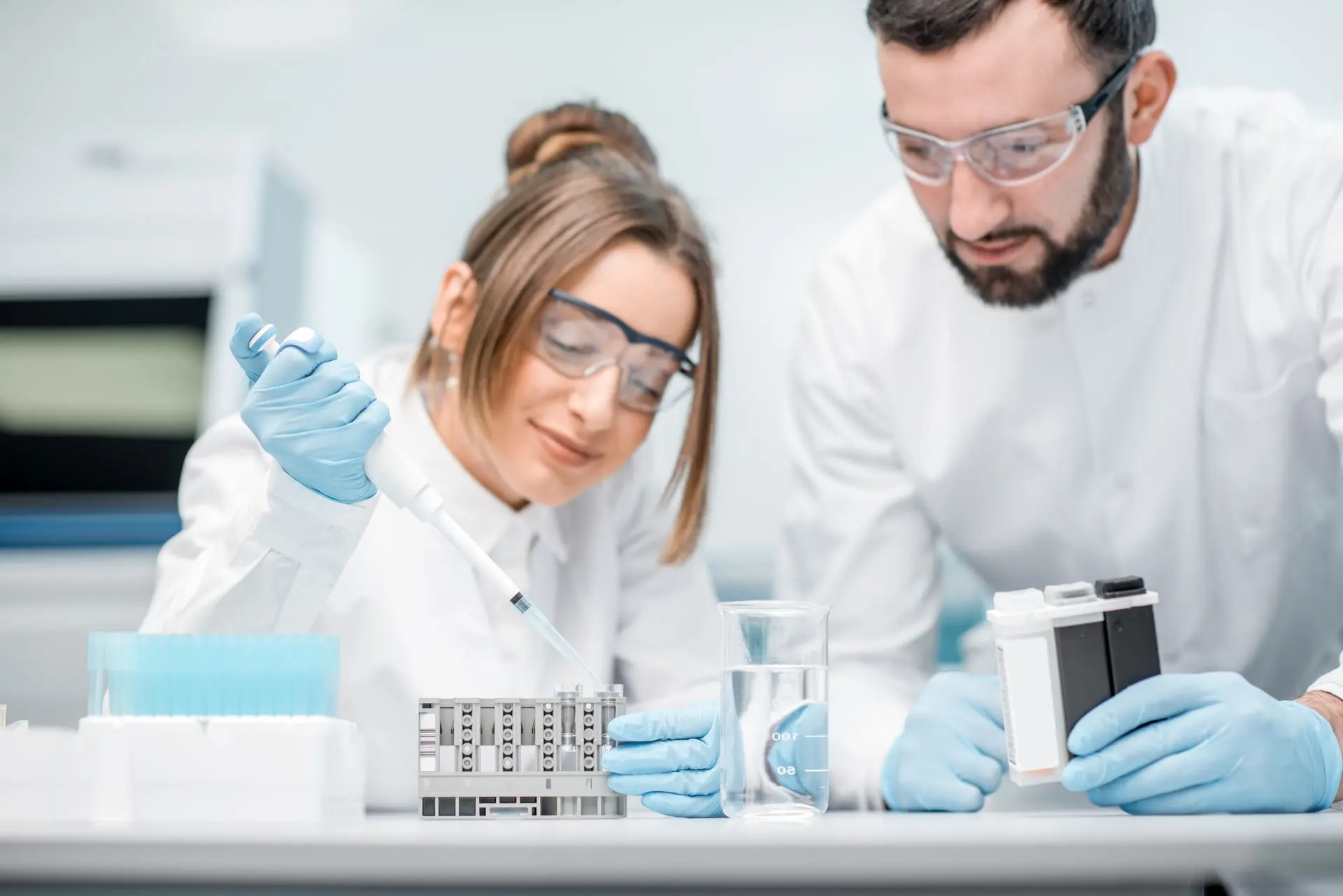
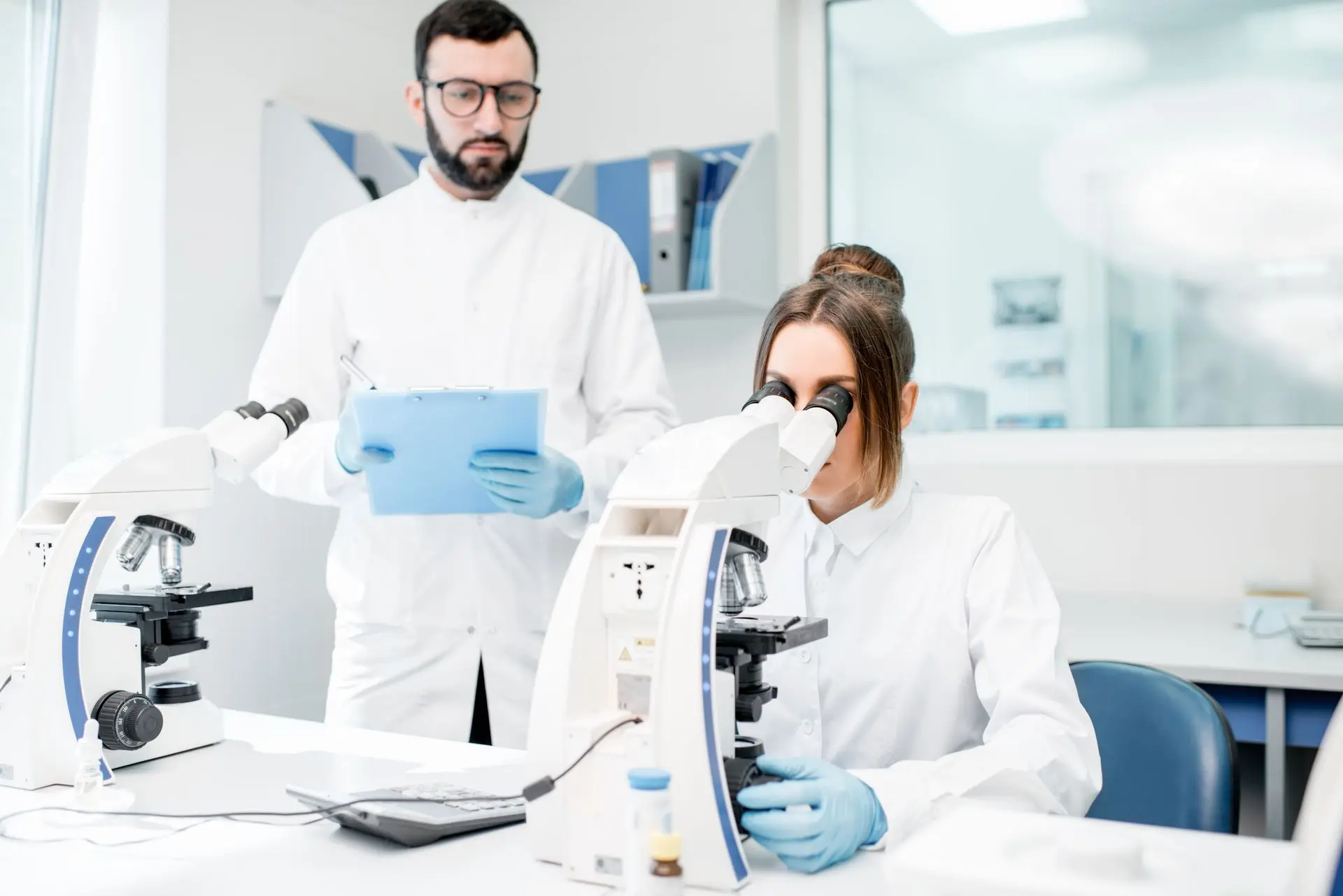
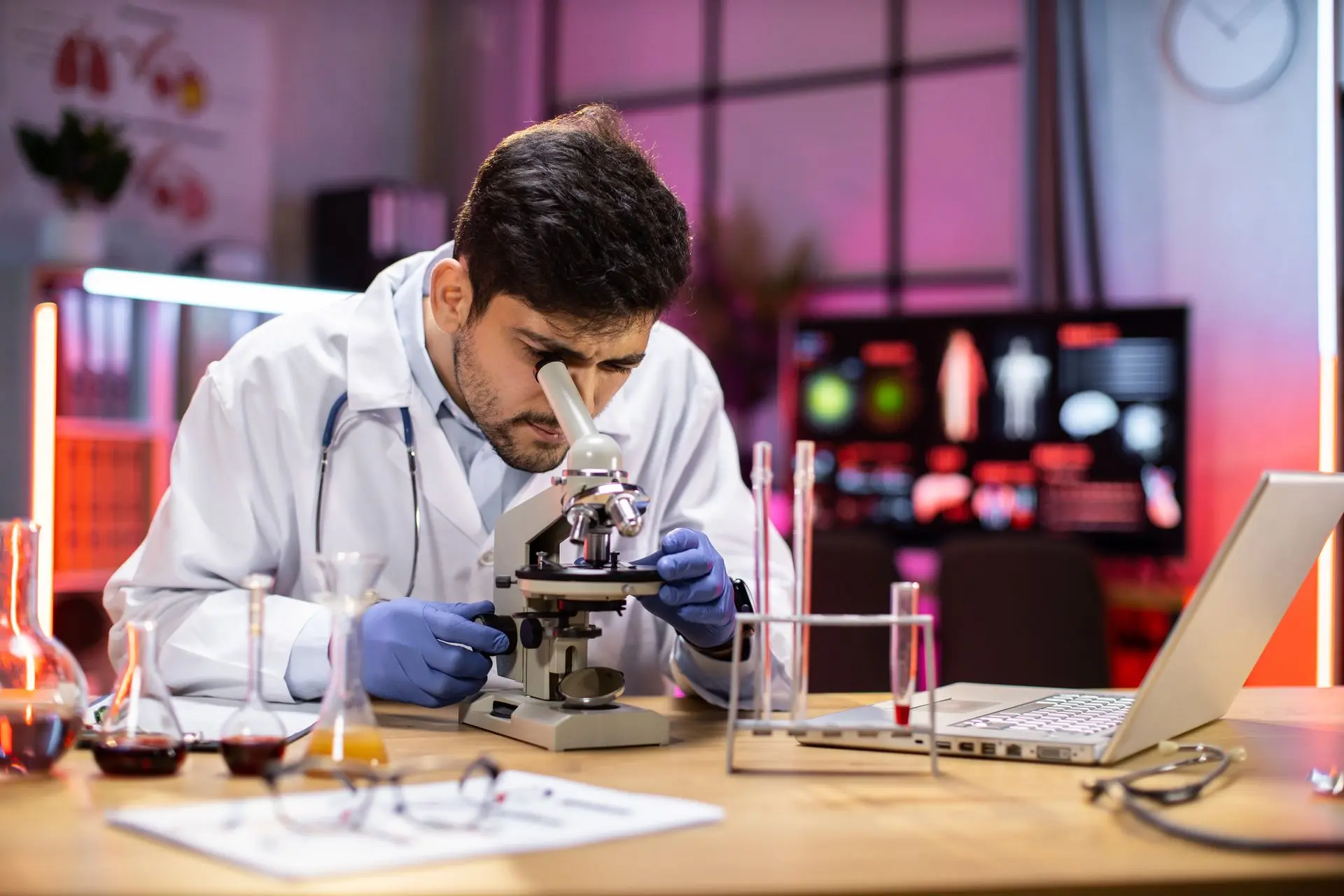
Resolve the neural computations that transform low-level visual representations into semantic content
Our novel methodological tools offer a unique integration of diverse data (MEG, fMRI, convolutional neural networks, and behavior) in a common RSA framework, enabling a holistic description of human brain function. This is fundamentally different than any available tools to date, and will enable novel experimental paradigms to test hypotheses in perception and cognition in the human brain.
To apply these tools, we have focused our efforts in a critical research area: human visual recognition. A multistage distributed network of cortical visual pathways provides the neural basis for object recognition in humans. While the computations and tuning properties of low-level neurons have been investigated in detail, the precise neural computations transforming low-level features to mid- and high-level representations remain terra ingognita. By operationalizing representations as similarities across pairs of stimuli in an RSA framework, we study the hierarchical cascade of the human visual system in a systematic way.
Bridge the gap between human and machine vision:
The past five years have seen considerable progress in using deep neural networks to model responses in the visual cortex. Deep neural networks (DNNs) are now the most successful biologically inspired models of computer vision, making them invaluable tools to study the computations performed by the human visual system. Recent work has shown these models achieve accuracy on par with human performance in many tasks. We have also shown that computer vision models share a hierarchical correspondence with neural object representations.
DNNs have adopted a feedforward architecture to sequentially transform visual signals into complex representations, akin to the human ventral stream. Even though models with purely feedforward architecture can easily recognize whole objects, they often mislabel objects in challenging conditions, such as incongruent object-background pairings, or ambiguous and partially occluded inputs. In contrast, models that incorporate recurrent connections are robust to partially occluded objects, suggesting the importance of recurrent processing for object recognition.
To continue bridging the gap between human and computer vision, we explore how the duration and sequencing of ventral stream processes can be used as constraints for guiding the development of computational models with recursive architecture.
Characterize function in the atypical brain, including neuroplasticity in sensory loss and pathologic activity in neurological disorders
We use our novel methodological approaches to study the atypical brain. The ideas in pursuing this goal are exemplified in the following projects.
Functional reorganization of brain representations in blindness :
The human visual cortex does not fall silent in blindness. Substantial neuroimaging and neurological evidence has shown that visual cortex activation in blind individuals is functionally relevant for nonvisual tasks (e.g. Braille reading, verbal memory, and auditory spatial tasks). Yet, the nature of these computations and the governing principles of functional reorganization in blindness remain elusive. The two main theoretical frameworks proposed so far posit opposing hierarchical organizations of representations. The co-opted hierarchy framework suggests that the visually deprived cortex processes information in a consistent bottom-up hierarchy similar to its role in visual processing. In contrast, the reverse hierarchy framework predicts that early visual cortex receives high-level content at the end of the processing cascade, with the processing hierarchy reversed compared to the typical brain. The overall objective of this project is to disambiguate between these two theoretical frameworks by constructing a finely resolved picture of the sensory processing cascade in blind persons. We will use the MEG-fMRI fusion method that will allow for the first time to capture the hierarchical neural cascade of Braille processing in blind individuals.
Variability in the auditory-evoked neural response as a potential mechanism for dyslexia
The goal of this project is to investigate the role of neural variability in dyslexia. In particular, we explore whether trial-by-trial neural variability differs in the auditory and/or visual cortex of children with dyslexia when compared to neurotypical children. Preliminary results indicate the autism condition is associated with decreased consistency in the neural response for both auditory and visual stimuli.
Sensitivity to speech distributional information in children with autism
This project investigates whether children with autism spectrum disorder are sensitive to probability cues in speech. In typical language acquisition literature, ample evidence suggests neurotypical children are exquisitely poised to capture the distributional information embedded in speech, to learn various aspects of phonotactic and syntactic rules. Children with autism, however, demonstrate impaired performance in such tasks. We use an auditory mismatch paradigm (syllables ‘ba’ and ‘da’ delivered with different probabilities) to detect deficits in probabilistic learning. Preliminary findings have revealed that impaired reading skills in autism are associated with atypical sensitivity to frequency of syllables.